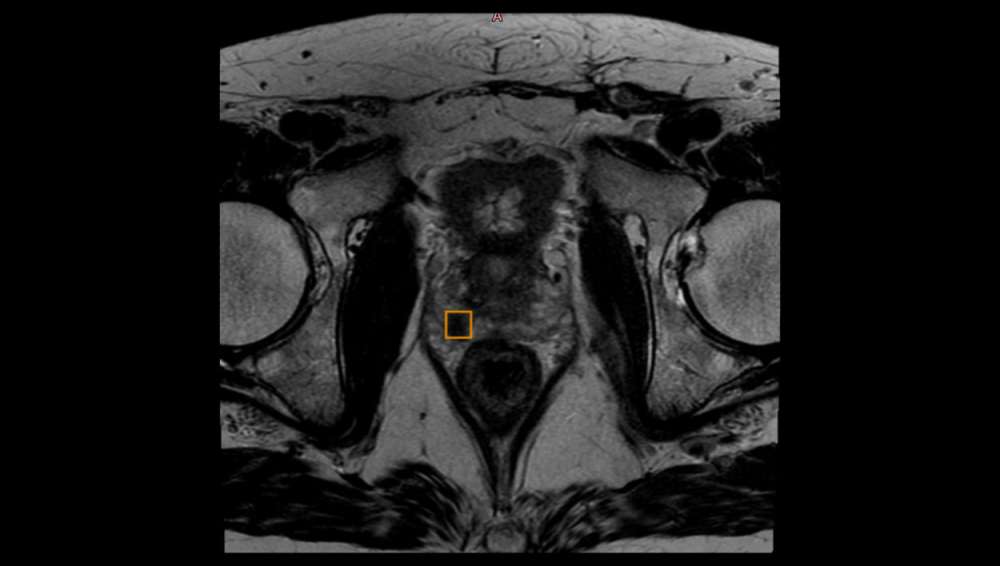
Picture by Quibim
QP-PROSTATE® – AI solution to increase diagnostic accuracy
Prostate cancer (PCa) is the second most common cause of cancer globally, as cited by the World Health Organization (WHO). Despite its high prevalence, our current cancer diagnostic tools for PCa often fall short, resulting in inaccurate
diagnoses. Clearly, we urgently need innovative tools to enhance accuracy in cancer diagnostic and better evaluate treatment responses. This is where the FLUTE project comes in.
The FLUTE project aims to sharpen predictions of aggressive prostate cancer while reducing unnecessary biopsies. This approach will significantly improve patient welfare and cut associated healthcare costs. The project will contribute to the global HL7 FHIR standard development and formulate new guidelines for GDPR-compliant cross-border Federated Learning in healthcare to maximize the results’ impact, adoption, and replicability.
Quibim, as a participant and experts on AI in healthcare, will contribute with the QP-Prostate® tool; an AI solution to increase diagnosis accuracy.
Quantitative imaging biomarkers (QIB) extracted by QP-Prostate® as input of the predictive models
QP-Prostate® is an AI-powered solution, which automatically evaluates imaging quality, segments the prostate gland and identifies suspicious lesions. Based on Convolutional Neural Networks (CNNs), QP-Prostate® automatically segments the three key subregions of the prostate gland: peripheral, transitional+central zones and seminal vesicles. Moreover, QP-Prostate® also automatically highlights regions suspicious for clinically significant prostate cancer (csPCa).
The AI algorithm has been trained with pathology outcomes as ground truth and it has been designed to efficiently detect the prostate cancer lesions using as input the biparametric sequences of magnetic resonance images (MRIs), being T2w and diffusion weighted imaging (DWI).
From the biparametric MRIs (bpMRI), QP-Prostate® provides the apparent diffusion coefficient (ADC) series, offering rich quantitative diffusion information. The ADC values have become an important biomarker in prostate cancer, since they provide information of the motion of water molecules in a free space. Pathological tissues are characterized for having a reduction in the interstitial space, causing a low diffusivity of the water molecules. Furthermore, if the dynamic contrast-enhancement (DCE) sequence is available, having a multiparametric MRI (mpMRI), the perfusion analysis is automatically generated, providing information of the following biomarkers: K trans , k ep and V e , which give information of the angiogenesis, indicating the tissue perfusion.
These quantitative parameters, as mentioned, provide meaningful clinical information, which will be used as input of the predictive model performed by another partner of the consortium, Gradiant. They, through machine learning, will use the segmentations and the quantitative imaging biomarkers maps of the prostate gland and lesions generated by QP-Prostate® from the baseline MRIs, as well as clinical data, for predicting the tumor aggressiveness.
Validation of the synthetic magnetic resonance images
Another objective of the project is the creation of synthetic images. Currently, access to health data is quite restricted due to security reasons, being difficult to have large amounts of data for the development of robust AI models. The generation of synthetic health data could help to alleviate this situation, at least in the early stages of AI model
development. In the frame of FLUTE project, the Universitat Politécnica de Cataluña (UPC), partner who leads this task, will develop novel methods of synthetic data generation using multimodal EHR data from hospitals and multi/biparametric MRIs. The bi and/or multi-parametric 3D images created will be validated by using the QIB maps extracted from QP-Prostate®.
Once the algorithm of synthetic images generation is validated, it will be integrated into the FLUTE platform. Hence, the innovators and researchers will be able to use synthetic data for model evaluation and for initial coarse training of models, fostering rapid digital healthcare innovation.
Álvaro Paricio Ruiz-Belloso, Mayra Rubio Martínez, Anna Nogué Infante